Image credit: © Orlando Ramirez-USA TODAY Sports
Baseball is back. On February 14, pitchers and catchers reported to Spring Training, and the reports of players being in the Best Shape Of Their Life have started pouring in. Absent from these festivities: the reigning National League Cy Young winner, who as of this writing is still unemployed. Part of that is certainly teams biding their time to maximize their leverage, but part of it may be that Blake Snell is one of the more difficult pitchers in baseball to project. Leaving aside his health record for a second, there’s some evidence of a weak foundation underlying his success. Others have written extensively about the concerns behind Snell’s league-leading 2.25 ERA last season, and they center around his propensity for giving hitters a free base. Consider how our Pitch Quality metrics, which value a pitcher based solely on the characteristics of his pitches and not on results, rank him among qualified starters: While his Stuff Quality ranks 13th among qualified starters, his Overall Pitch Quality—which takes into account both Stuff and Locations—ranks 36th. Put simply, he’s not hitting good enough locations to maximize the value of his stuff.
Where analysts begin to disagree is in what’s driving such seemingly poor location of his pitches. While many attribute his walks to poor command, others have argued they are by design: a worthwhile by-product of targeting the edges of the zone and being unwilling to give in and risk hard contact. The truth is likely some combination of both, but it’s difficult to disentangle the two without knowing where Blake was targeting with each pitch. Luckily for us, a presentation at last year’s Saber Seminar by Scott Powers and Vicente Iglesias offered a roadmap for doing just that. They used what’s known as a Hierarchical Model to estimate the average and standard deviation of a pitcher’s pitch locations and then simulate new locations using these model estimates. Our methodology differs slightly from theirs, and though it may sound complicated, the general approach is intuitive and easy to follow.
We start by modeling the expected horizontal and vertical location of each pitch based on the type of pitch it was, the count in which it was thrown, the handedness of the batter and pitcher, and the identity of the batter. Next, we adjust these expected locations based on the general tendencies of the pitcher.[1]
These expected locations are treated as the pitcher’s target for a given pitch. Using these target locations and the actual observed locations of each pitch, we are then able to model the average and standard deviation of the distance and direction of each pitcher’s misses. These average miss distances and locations are extremely sticky, with a year-to-year correlation of 0.7 among pitchers with at least 500 pitches thrown. For comparison, the year-to-year correlation of a pitcher’s strikeout rate is around 0.6.
Now that we have all of these expected locations and spread in locations for each pitcher, we can simulate new pitches using different assumptions. First, let’s simulate a bunch of fastballs from Blake Snell using his own average target location, miss distance, and miss direction and plot them together with his actual fastball pitch locations.
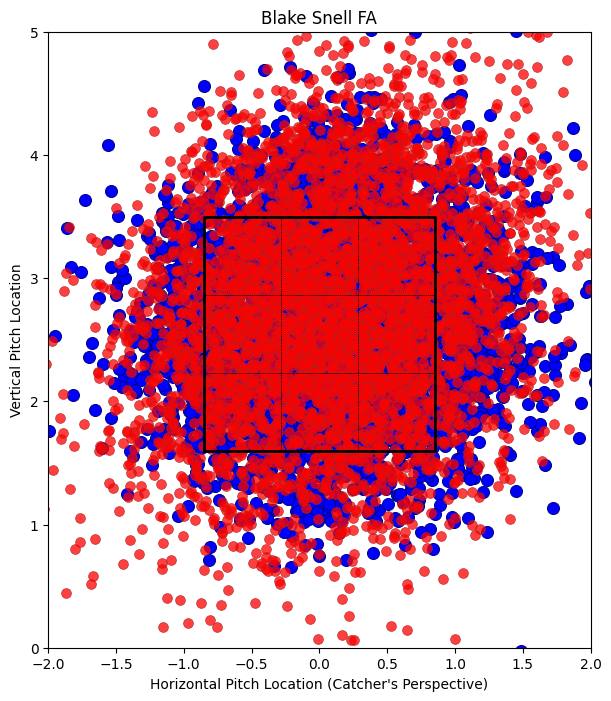
I chose not to label which pitches were real and which were simulated for a reason. Can you tell the difference between them? Because I sure can’t.[2] Next, let’s simulate another set of fastballs, but this time we’ll use an average pitcher’s spread in miss distances to see how that compares to his own spread.
Here I’ve plotted the simulated pitches from Snell’s own miss distribution in blue and from an average pitcher’s miss distribution in red. Note just how many more pitches end up out of the zone (often far enough out to qualify as waste pitches), indicating that regardless of where Snell is targeting, he’s struggling to hit his spots relative to an average pitcher in the same scenarios. And it’s not just his fastball. Among 479 pitchers who threw at least 500 pitches in 2023, Blake Snell’s average miss distance ranked 425th. Score one point to the command camp. But before we declare a winner, let’s also look at his expected target locations.
This time we’ll look at Snell’s slider, and instead of a scatter plot of individual pitches we’ll plot a contour of his locations with a marker for his typical likely pitch target and where an average pitcher would have targeted those same pitches given the context.
That roughly six-inch difference in targets takes a number of what would be well-located chases down and away and turns them into easy takes for a ball. Sure, it may save him from some hard contact but, when your slider generates whiffs as well as his, I see no reason why he should be wasting them out of the zone. He should be challenging hitters and daring them to put a barrel on it.
So we’re back to where we started, with both the “poor command” and the “poor approach” camps having plenty of evidence for what led to Snell’s abundance of walks, albeit we now have the pretty plots to back each side up. The natural follow-up is: Which is hurting the value of his pitches more? To evaluate this, we can feed all of our simulated pitches into our Pitch Quality model. We’ll start by simulating a full season of pitches from Snell using his own targets and miss characteristics. Then we’ll simulate a season using his own targets but an average pitcher’s miss characteristics. Finally, we’ll simulate a third season using his own miss characteristics but an average pitcher’s target for each pitch. The differences between the models should reveal whether his command or his approach was having a larger impact on his expected pitch value.
Our modeled simulations find that if Blake Snell were to improve his command to that of an average pitcher, the expected run value of his pitches would improve by a little more than half a run per 100 pitches. If instead he were to keep his same command but simply target areas more typical of an average pitcher, his expected run value would improve more than three-quarters of a run per 100 pitches. The command may be bad, but the approach appears to be even worse.
To understand why the models show such discrete differences in predicted value, we can look deeper into the predicted outcomes of each pitch from the separate models. Below I’ve added a column for the predicted difference in value of balls in play, difference in swing rate, and difference in the number of balls thrown between each of the models.
To others’ points, a change in command or in approach for Snell would leave more balls over the middle of the plate, leading to both more contact and more hard contact. Over a full season, the model believes that this would cost Snell nearly 9 runs in value if he had average command. However, this impact is swamped by how many fewer balls he would throw due to the change, resulting in both fewer walks and fewer favorable counts for the hitters.. The model found that a change in command would result in an increase in swing rate of eight percent and a decrease of 361 balls thrown. For a change in targets the differences were four percent and 205, respectively. He’s better off attacking the zone and accepting the occasional hard hit ball than he is nibbling and racking up walks.
The question for a team looking to sign Snell is twofold. One, is there some value to his approach that our Pitch Quality models are missing? Given the impressive correlation between the model predictions and the observed outcome of pitches, we find this unlikely. Which leads to the second question: is Snell open to a change in approach? If Snell were an up-and-coming rookie it may not be much of a question. But we’re talking about a 31-year-old established veteran with two Cy Youngs under his belt. I can’t say that I would be amenable to changing a single thing were I in his shoes. Maybe his raw stuff will continue to carry him, but velocity doesn’t last forever. At some point, Blake Snell is going to need to make a change, and it starts with throwing strikes.
[1]For the nerds: I used MAP estimates of the mean from a multivariate-normal hierarchical model in pymc. The model was structured such that global means were estimated for each combination of pitcher handedness, batter handedness, pitch type, and count. Then, individual means were estimated for each batter and each pitcher ID based on pitch type, platoon advantage, and count.
[2] Blue dots are simulated.
Thank you for reading
This is a free article. If you enjoyed it, consider subscribing to Baseball Prospectus. Subscriptions support ongoing public baseball research and analysis in an increasingly proprietary environment.